Data Science, Optimization, and Sustainable Development
In the year 2015, United Nation member states proposed a blueprint for a better and sustainable world named Sustainable Development Goal (SDG) with 17 goals and 169 targets which need to be accomplished by the year 2030. One of the major challenges is tracking the development to visualize the progress towards sustainability. The problems are more severe in developing and poor countries due to lack of reliable data sources. Our aim is to track the principal goals such as Climate Action (Goal-13), Food Security (Goal-2), Poverty (Goal-1), Water Security (Goal-6), Clean Energy (Goal-7), Health (Goal-3) and Education (Goal-4) using machine learning and remote sensing (as the satellite data are hugely available). We intend to develop a better machine learning algorithm which will incorporate historical and satellite data to track those goals with high accuracy. Though the satellite data is highly unstructured & noisy but we are trying to cope with the challenges.
More crops whilst saving drops using a linear programming optimization model
- Karnafuli Irrigation Project (KIP) is one of the most contributed irrigation projects in Bangladesh, which is established with an objective of an increase in crop production by about 35,000 tons. But, KIP is losing its performance due to low irrigation efficiency. This research determines the optimal use of irrigation water and irrigable area in the Karnafuli Irrigation Project of Bangladesh to maximize crop production and, subsequently, the net benefit considering climate change effects by developing an optimization model using linear programming. Increased crop production with available water drops ensures both food and water security. This study also contributes to the Food and Agriculture Organization (FAO) and the International Water Management Institute (IWMI) ‘more crops per drops’ theme. [Paper]
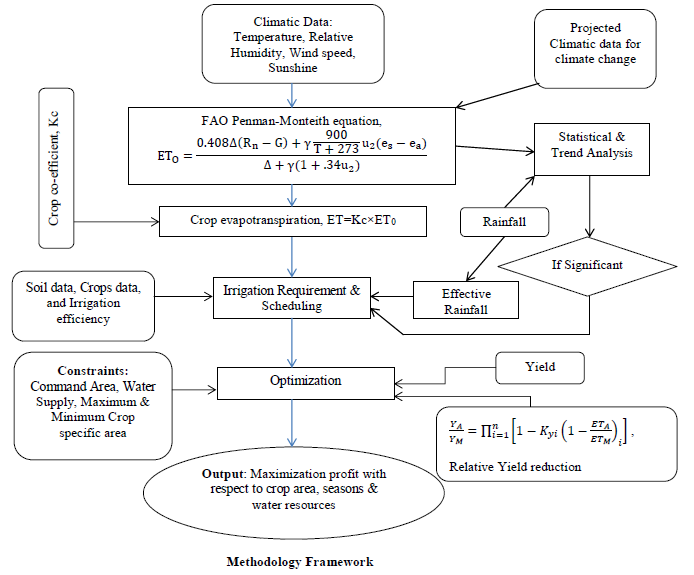
Deep unsupervised sensor calibration improves crop classification
- Here, I am combining deep learning, remote sensing, and computer vision to train high-resolution satellite imagery for a better crop monitoring system.